Generative AI has the spotlight right now, but that doesn't mean machine learning is slowing down. In fact, machine learning is expected to show an annual growth rate of 18.73%, resulting in a market volume of US$528.10bn by 2030.
With this kind of growth, new applications for machine learning in marketing will emerge. The marketers who jump on early and unlock the data-driven insights machine learning can offer will come out on top.
What is machine learning?
Machine learning is a subset of artificial intelligence. Its market definition, according to Statista, is "the use of algorithms and statistical models to enable computer systems to learn from data and improve their performance on a task."
Machine learning algorithms can analyze huge volumes of data to discover patterns that humans would struggle to find themselves. Reporting on performance, generating insights for optimizations, and using predictive analytics to forecast outcomes or resource requirements are all well-known applications of machine learning.
Machine learning vs artificial intelligence marketing?
Artificial intelligence in marketing will use both offline and online customer data alongside AI technologies (including machine learning) to analyze information, predict audience behavior based on actions or societal and economic trends, and automate marketing campaigns, customer support responses, digital ad buying, lead scoring, and that’s just the beginning.
Machine learning in marketing is the data collection and analysis used to help make the forecasts for the AI. The algorithms used by machine learning, work to study the data provided to then be able to perform actions, resulting in better outcomes. It helps marketers be able to optimize and improve the customer journey by making data-driven decisions, and ultimately improve customer experiences.
5 fast facts
Early adopters of machine learning saw a 47% improvement of their sales and marketing efforts. (Deloitte)
There are close to 100,000 jobs worldwide requiring machine learning. Nearly half of them are based in the U.S. (Forbes)
Netflix saves up $1 billion per year due to machine learning. (Lighthouse Labs)
Of the surveyed customers, 62% do not have a problem in sending their usage data to an artificial intelligence platform to improve machine learning if it results in an improved customer experience. (Salesforce)
48% of businesses use machine learning, data analysis, and artificial intelligence tools to maintain data accuracy. (O’Reilly)
How machine learning can help marketers today
Without machine learning, it would be increasingly difficult for marketers to compile, absorb, and analyze the vast streams of data coming from multiple sources, let alone generate meaningful insights or make predictions on future campaign performance.
Here are a few more ways machine learning can help marketers:
1. Analytics
Large data sets are where machine learning programs thrive. It can easily sift through huge amounts of data in a short amount of time to spot complex patterns and predict probable outcomes.
As a marketer, the insights generated from machine learning can help you better understand your customers, their journey, how your campaigns are performing, and more. It can also help you forecast leads or revenue, and predict how certain content pieces will perform.
With machine learning models, you can do all this at scale, and at speed. This is essentially what makes it possible to be a data-driven marketer in an incredibly fast-paced and competitive landscape.
3. Automated A/B testing
A/B tests help you test and learn pieces of content like email subject lines, SMS content, ad copy, and more. They're a great way to optimize a campaign's performance while learning more about the kind of messaging that resonates with your audience.
Machine learning is what allows you to automate these tests. The algorithm assigns the control and treatment group, sends each a different variant of the content, then collects data on the outcomes and uses it to identify which is the best-performing option. There are variations on this experiment, some of which are more complex,
4. Customer segmentation
Customer segmentation is an essential part of marketing today. It's what enables marketers to scale personalization, and better understand the audience they're speaking to.
Machine learning ensures that your segmentation efforts are both accurate and efficient. This means that you can target a very specific segment of your audience with a message at any time, without waiting for your data team to run an SQL query to find the right segment.
5. Forecast and predict sales outcomes
There's a machine learning algorithm that underpins your CDP or CRM to help you score leads and forecast revenue. It's a crucial part of setting relevant and realistic goals, and accurately predicting things like inventory and staffing requirements in the future.
Marketers can also use this information to assess the quality of the leads certain campaigns and activities are bringing in and make more accurate predictions on the ROI for a campaign. This can help you set budgets, justify spend, and make better decisions about which advertising platforms to spend with.
Final word
Without machine learning, much of what data-driven marketers do in their day-to-day would be impossible. Today, the algorithms at play are becoming more and more sophisticated, enabling more accurate predictions and automated optimizations.
First published April 2022, updated for relevancy December 2023
Author
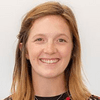
More by Meagan Philpot
Meagan Philpot has no more articles