Great marketers today are data-driven marketers. Marketers are expected to shape their go-to-market strategy based on the data they have on leads and customers and use it to personalize and optimize campaigns, and measure performance.
This doesn’t mean every marketer needs to be a data scientist — but it does mean marketers need to have a base-level understanding of the data they have and how they can get the most out of it.
Part of this is understanding the different types of data — data-driven marketing strategies rely on both structured and unstructured data, for generating insights, segmenting your audience, and personalizing your marketing messages.
In this article, we’ll explain what structured and unstructured data is, the benefits and challenges each type presents, how marketers can leverage each, and the role AI and machine learning can play in helping marketers unlock the power of their data.
What is structured data?
Structured data is a data set that lives within a standardized format with a clear and well-defined structure. It consistently complies with a data model and can be easily understood and searched by software and humans.
It’s the type of data most marketers will be most familiar and comfortable with because it is orderly and easier to search, organize, and use.
Examples and applications of structured data in marketing
Customer databases (CRM or CDP)
Demographic, firmographic, and behavioral data in your CRM or CDP is structured, organized, and consistent. Common applications for this type of data include audience segmentation, lead analysis, and building ideal customer profiles.
Website analytics
Views, sessions, time spent, and other structured website analytics data are available to marketers in a number of platforms, most commonly GA4. It can be used to analyze performance and optimize website performance.
Sales and marketing metrics
Metrics from sales and marketing including revenue figures, lead volume, and campaign engagement metrics (for example, email opens or click-through rates), are most commonly used for performance analysis, to spot trends and generate insights.
Advantages and disadvantages of structured data
The major advantage of structured data is that it is easy to analyze and work with.
Whether your structured data is stored in a CRM, CDP, or elsewhere, it is typically easy to integrate with marketing automation tools, making it easy to use in personalized and behavior-based campaigns.
If you are drawing insights from your data or analyzing performance, structured data gives you comparable data points that can be used to identify trends or measure against KPIs.
This benefit also has its drawbacks. Structured data is often organized and controlled in a way that makes it difficult to use for unintended purposes. This can limit its flexibility and can prevent marketers from using it in unique ways.
What is unstructured data?
Unstructured data is data that is not organized to a preset data model or schema. It does not follow a specific pattern or defined structure, making it difficult to store in a database format. This complex, diverse data type can be difficult to understand and analyze, but it can offer deeper insights for marketers who want to better understand their customer’s behavior.
Most businesses have huge amounts of unstructured data at their disposal, but its disorganized nature makes it very difficult to work with.
Examples and applications of unstructured data in marketing
Communications including call transcripts, meeting recordings, chat, and instant messages
Communications data be used to assess lead sentiment and likelihood of converting, in performance analysis, or to spot trends in questions
Social media posts from LinkedIn, Instagram, and others
Data from social posts can be used for social listening, sentiment analysis, and to identify rising consumer trends and behaviors
Customer feedback on review sites, social platforms, and feedback forms
Review data can be useful to spot overarching sentiment trends and better understand your ideal customer profile
Email and SMS messages (though email is sometimes considered to be ‘semi-structured’ - more on that later)
Data from email and SMS can be used to analyze sentiment, generate frequently asked questions and answers, track support performance, measure replies on marketing campaigns, and understand customer emotions in different contexts.
Advantages and disadvantages of unstructured data for marketers
Unstructured data is particularly useful for assessing brand sentiment and understanding how your leads and customers react to your brand or product on an emotional level. People are often more driven by an emotional pull towards a brand than a logical one, so understanding trends and picking up on any peaks or pits in sentiment around campaigns or initiatives can help marketers understand what’s working beyond the direct response metrics.
This kind of data can also be helpful for research and development, particularly when it comes to problems your customers or leads are facing that could be solved with new product innovation, service offerings, or improvements to your existing product or services.
The disadvantage of unstructured data is that it can be difficult to work with and analyze at scale. Most businesses have huge amounts of this data, making the disorganized nature of it even more prohibitive.
Thankfully, tools that use machine learning and artificial intelligence have made working through this large volume of unstructured data possible. Social listening, brand sentiment, and sales intelligence tools are three commonly-used examples of this.
What is semi-structured data?
There is an in-between that exists with data — semi-structured data. This includes data types that combine structured and unstructured elements. As mentioned, email falls into this category. This is because email contains both unstructured data (the contents of the email including text and media), and structured data in the form of metadata like sender and recipient, route, timestamp, content type, and DomainKeys Identified Mail (DKIM).
Other examples of semi-structured data include survey results and feedback forms that combine structured responses (multiple choice or drop-down response fields) as well as unstructured responses (open-ended fields).
Semi-structured data in marketing is helpful because it has some elements that can be more easily understood and organized, and others that offer deeper insights.
Four key differences between structured and unstructured data
To recap, there are four key differences between structured and unstructured data for marketers.
Sources
Structured data is sourced from online forms, network logs, website logs, tracking pixels, online transactional processing (OLTP) systems, and more. Unstructured data comes from messaging channels, documents, social media platforms and some open-ended online form fields.
Format
Structured data consists of only numbers and values in a predefined data model that is comparable and consistent. It tends to be organized before it is stored in a platform like a CRM, CDP, or analytics tool. Unstructured data can include varied types (text, images, video, audio) and is stored in its native format. It is not processed until it is being used.
Storage
Structured data is stored in tabular formats, like sheets or an SQL database. Because of this, it requires less storage space and can therefore be stored in data warehouses. Unstructured data is stored as media files, requiring far more space and therefore requiring a data lake for storage.
Use cases for marketers
Structured data helps marketers measure performance and personalize communications, it is also used for machine learning algorithms on ad platforms and elsewhere. Unstructured data is used in natural language processing (NLP), text mining, and by data scientists and AI tools to provide insights into customer sentiment and brand perception.
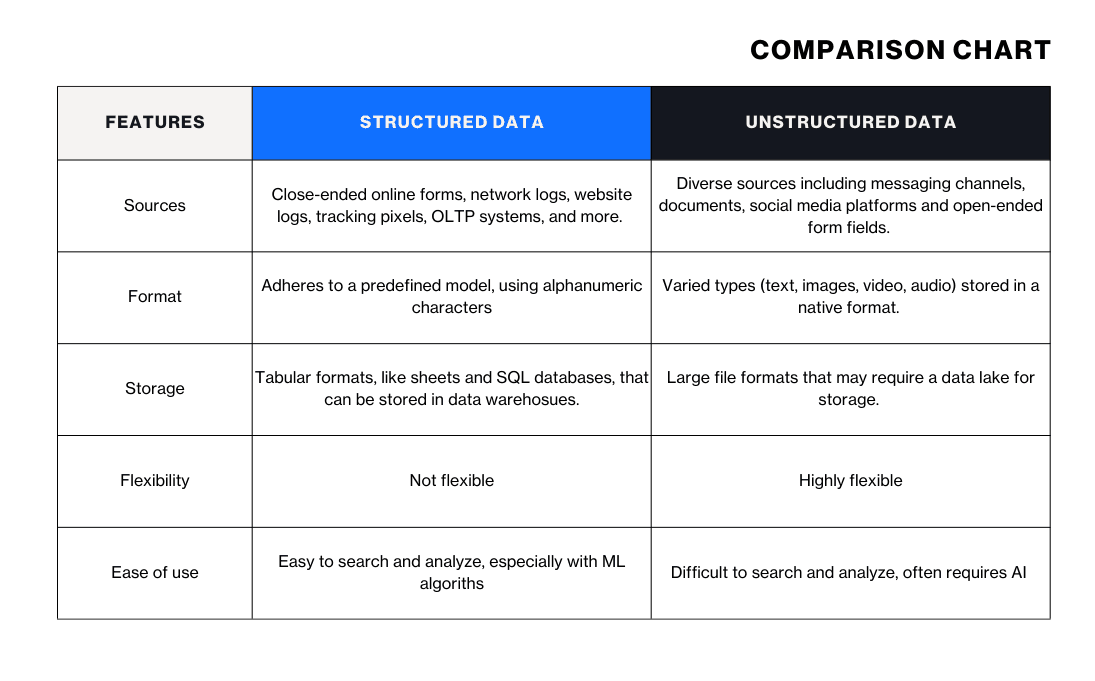
The future of unstructured vs. structured data
It wasn’t too long ago that the conversations around ‘big data’ were centered on the difficulty of analyzing and making good use of large and often unstructured datasets. Now, thanks to huge advances in AI and machine learning technologies, this conversation has changed for the better.
Unstructured, structured, and semi-structured data can all be processed at scale with the help of AI. This means that marketers can successfully do things like:
Analyze sentiment analytics and spot trends in communications and social posts: AI and machine learning technologies are great at pattern recognition. This means they can help marketers search through unstructured email, SMS, social, and chat data to do things like identify trends and measure customer sentiment.
Predict customer behavior: AI can mine customer data to spot patterns and predict future behavior. This can help businesses identify customers who are at-risk of churn, and more accurately forecast expansion revenue.
Real-time campaign optimization: When large volumes of data can be processed quickly, AI and machine learning algorithms can identify which campaigns in ad platforms are performing best, and optimize towards them. This can also help with things like email and SMS testing.
Identify opportunities: Unstructured data from social, email, and chat platforms can be used in conjunction with structured data from surveys and product usage or purchase information to identify unmet customer needs, requests from leads, and other growth opportunities. This information can later help marketers identify the best lead and messaging for launches.
Optimize resources: Your data can help you identify where you have excess or untapped resources, skills gaps, unused software or applications, and seasonal spikes that may require freelance help. Using this data to minimize operational costs while maximizing output can help improve your bottom line.
As AI is more readily adopted by marketers, new use cases will emerge along with advancements in the technology. The potential here is huge for performance-focused and data-driven marketers.
Structured and unstructured data: Optimizing your data
Both structured and unstructured data are essential for marketing. They play different roles — with one being easily accessible and used for simple personalization, reporting, and analysis, and the other available for deeper analysis into sentiment and behavior.
Marketers who have an understanding of the types of data they have available and how they can use it are better placed to optimize campaigns, create efficiencies, identify opportunities, and speak directly to their leads and customers.